Natural language generation
Can Megatron-Turing NLG assume the role of our intelligence watchdog?
As we face more significant conflicts in geopolitical unrest, climate crises, and grand-scale financial impropriety, having tools in place to keep our intelligence agencies on a righteous path is more crucial than ever.
Who can we trust to watch the watchers? Who can we expect to be more intelligent than intelligence agencies?
Countries entrust intelligence organisations with doing what’s right for the greater good. Yet, it’s natural for that type of power to corrupt certain people. It’s not like these agencies are hiring robots programmed to only do what’s noble and just.
The fact is, we live in the information age, meaning everybody is under scrutiny. Unethical and criminal behaviours at high levels are under a microscope. People don’t trust authority figures and governing bodies as they once did. For example, just over 50% of American citizens still trust that US intelligence agencies protect their best interests. While we’re in the UK, sentiments from North America can often mirror our own.
Thus, the heightened focus on the intersection between intelligence agencies and corruption is unsurprising.
While public naivety regarding the behaviours of intelligence agencies is at an all-time low, that doesn’t mean it’s easy to catch bad actors.
As we face more significant conflicts in geopolitical unrest, climate crises, and grand-scale financial impropriety, having tools in place to keep our intelligence agencies on a righteous path is more crucial than ever.
One of those tools might be the Megatron-Turing Natural Language Generation Model (MT-NLG). Below, we’ll examine the nature of this innovative technology and how it can impact intelligence on a grand scale.
One NLG use-case example is incorporating it into customer input analysis. This enables organisations to offer personalised, concise responses.
Exploring Natural Language Generation
Before getting into the specifics of MT-NLG, let’s first examine the concept of Natural Language Generation and its broader implications.
A software process spearheaded by AI, NLG produces natural spoken or written language from data (which can be structured or unstructured).
One NLG use-case example is incorporating it into customer input analysis (e.g., voice assistant commands, chatbot queries, survey form feedback, or help centre calls). This enables organisations to offer personalised, concise responses and is why chatbots and voice assistants can seem human.
Furthermore, NLG-based tech can transform complex data–like numerical data input–into easily digestible reports. Such tools can generate automatic weather updates, financial reports, etc.
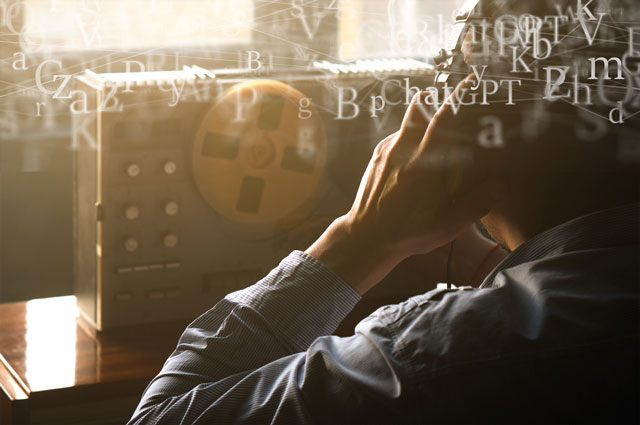
What are the nuts and bolts of NLG?
Here are some of the functional components of NLG:
Computational linguistics
- This component involves the scientific grasp of spoken and written language from a computer-based analysis perspective.
- Written/spoken dialogue must be broken down.
- A system must be created so that computer software can use the dialogue.
- Semantic and grammatical frameworks help generate a language model system, enabling accurate speech analysis.
Natural Language Processing (NLP)
- Computational linguistics is applied to human language (either spoken or written).
- NLG is actually an NLP subcategory.
Natural Language Understanding (NLU)
- This NLG component attempts to ascertain a speaker’s emotion, effort, intent, and goals behind their communication.
- NLU enters a deeper level of machine learning for more intricately detailed comprehension.
We’ve taken our deep dive into one aspect of MT-NLG. Now, it’s time to investigate the other puzzle pieces.
Unlike models from previous generations (e.g., BERT), the residual connection and layer normalisation position in the model architecture are swapped. This enables continual improvement in the models as they scale upward.
What is Megatron-LM?
Megatron-LM is a massive, dynamic transformer innovated by NVIDIA’s Applied Deep Learning team.
Unlike models from previous generations (e.g., BERT), the residual connection and layer normalisation position in the model architecture are swapped. This enables continual improvement in the models as they scale upward.
With higher NLP task scores than BERT, Megaton-LM offers increased details on model-parallel, efficient, and multi-node GPT and BERT pre-training.
Megatron-LM functions as an efficient, highly optimised library that trains large language models. It offers model parallelism to train language models with billions of weights. It then incorporates NeMo for downstream tasks.
NeMo automatically manages Megatron-LM pre-trained model parallel checkpoints. NeMo-based parallel models mirror the features of other NeMo Models.
What is Turing NLG (T-NLG)?
Microsoft-developed T-NLG is a generative language model based on a transformer. It generates words to complete open-ended textual tasks, completes unfinished sentences, and produces direct answers to input document questions and summaries.
This tech enables users to respond as fluently, accurately, and directly as humans in all situations. Systems no longer have to rely on existing content extraction from stand-in documents to provide answers. T-NLG does so naturally for email threads and personal documents.
MT-NLG has 3-times the parameters of its closest counterpart, providing unparalleled accuracy.
Putting all the pieces together
Microsoft and NVIDIA have combined to develop the Megatron-Turing Natural Language Generation Model. It’s the largest and most dynamic monolithic transformer language model in existence, boasting 530 billion parameters and a significant advancement in AI-based natural language generation.
MT-NLG has 3-times the parameters of its closest counterpart, providing unparalleled accuracy and the following natural language tasks:
- Reading comprehension
- Completion prediction
- Natural language inferences
- Word sense disambiguation
- Commonsense reasoning
How can MT-NLG be an intelligence watchdog?
You can scour the internet for articles about MT-NLG, and you won’t find much about its potential role as an intelligence watchdog.
Instead, we have to look at the capabilities of such a tool and figure out how it can play a vital role.
First and foremost, keeping a watchful eye on intelligence agencies means being able to parse through complex communications. It requires the ability to read emotions, delve into ambiguities, and make inferences based on things that have been spoken or written.
Humans–specifically, trained experts–have the power to do this independently.
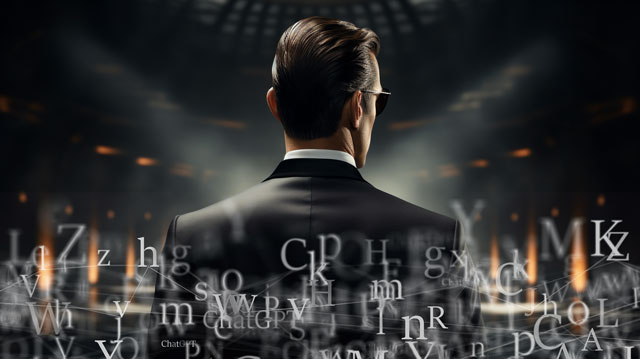
Cases that take decades to make may only take a few years, with a tool like MT-NLG helping investigators flag potential impropriety in intelligence agencies.
Sure, espionage movies like James Bond and the Bourne series are dramatizations, but they come from somewhere. Malfeasance and corruption from within are common themes, and people–both external and internal watchdogs–have policed and investigated these actions for generations.
Nonetheless, utilising tools like MT-NLG can help superpower these efforts.
After all, uncovering the wrongdoings and misdeeds of bad actors in these settings requires evidence and document analysis. MT-NLG can help human watchdogs do this on a grand scale, with previously inconceivable efficiency. Cases that take decades to make may only take a few years, with a tool like MT-NLG helping investigators flag potential impropriety in intelligence agencies.
Only time will tell, though.
The future will tell the tale of MT-NLG’s role in intelligence
Undoubtedly, the capabilities of MT-NLG and all its potential use cases are astounding. The changes it can make to this world, especially when leveraged by people in industrious ways, can be much to the betterment of societies.
As for MT-NLG’s place as an intelligence watchdog, the possibilities are highly intriguing. At the very least, it’s already a reason for bad actors to begin sweating.