insight
Can bias truly be eradicated from AI?
Concerns about biases in AI raise questions about its ability to make the positive social impact it could and should.
It’s impossible to illustrate the potential benefits of AI in a few sentences. Broadly speaking, we know it will aid society–businesses and people–by automating repetitive processes. We also know AI will bolster productivity, complex problem-solving, and decision-making.
AI technologies are finding themselves in increased demand in a world that calls for immediate solutions combined with a high standard of quality.
Due to the ubiquitous nature of AI’s benefits, the related technologies are projected to completely shift dynamics in a long list of industries (if they haven’t done so already). These sectors include:
- Cybersecurity
- IT
- Logistics
- Healthcare
- Finance/Financial Services
- Agriculture
- Transportation
- Education
- eCommerce
- Entertainment
- Advertising
Plus, the lifestyles of individuals are bound to change due to the advent of AI technologies. The recent mainstream adoption and implementation of ChatGPT is a cultural tipping point. The tool has proved itself genuinely useful for many tasks, from developing software to brainstorming legitimate business ideas to writing a keynote speech.
Despite its many benefits, AI (specifically, tools like ChatGPT) presents many conundrums, conflicts, and ethical quandaries.
While other iterations of ChatGPT existed before, the current quality output is much higher...to a sometimes staggering point.
Despite its many benefits, AI (specifically, tools like ChatGPT) presents many conundrums, conflicts, and ethical quandaries. Undoubtedly, AI is the wave of the future. It can’t be avoided at this point, and that should be a good thing.
After all, AI can make the world a better place.
Yet, there remain blindspots and concerns with various aspects of this game-changing tech. For instance, concerns about biases in AI raise questions about its ability to make the positive social impact it could and should.
Examining the nature of AI bias
There’s a notion that machines can’t possibly be biased. Humans, not computers, are the ones with emotions and inclinations to lean in one direction or another based on lived experiences.
Yet, there are growing reasons to believe that AI makes choices that aren’t systematically fair to specific communities. As such, many researchers cite biases in AI that can potentially cause significant societal harm.
So how can something as seemingly objective as technology carry inherent biases?
The answer is straightforward: human beings.
People select the data used in algorithms. They also decide how to apply algorithm-generated results.
Diverse teams and extensive testing are required to mitigate unconscious biases in machine learning models. This way, AI systems are less likely to automate and project biased models.
Whether team diversity or rigorous tests can 100% eliminate biases instead of reducing them is a different question and one we wish to explore throughout this article.
If men are the only ones clicking the ads for high-paying roles, the algorithm will adapt and show them strictly to males.
Examples of AI bias
Below, we’ll examine some specific examples of bias in AI:
Presenting CEOs as males
We’ll look to our friends in the US as we examine AI bias examples, starting with how the related tech favours male CEOs over females.
While 27% of US CEOs are females, a study found that 11% of individuals appearing in Google picture searches for “CEO” were women.
Independent research conducted a few months later by Carnegie Mellon University discovered how Google’s online advertising systems displayed high-paying positions to females less than males.
Google spokespeople explained how advertisers can dictate who stumbles across their ads. Gender is a specification these organisations can set.
Moreover, a belief exists that user behaviours influenced this result. For instance, if men are the only ones clicking the ads for high-paying roles, the algorithm will adapt and show them strictly to males.
Racial biases in healthcare
When trained on non-representative data, AI systems often perform poorly for underrepresented US populations.
Studies from 2019 discovered US hospitals were using an algorithm that significantly favoured white patients over patients of colour when predicting patients requiring additional care.
The issue arose because the AI worked off the assumption that healthcare expense amounts accurately reflected individual healthcare needs. In reality, researchers found that patients of colour spent less on healthcare than white patients who shared similar conditions.
It’s worth noting that the bias was eventually reduced by 80%. However, that means some bias remained.
Facial recognition biases
One study discovered that facial recognition AI frequently misidentifies people of colour. The pitfalls here go beyond concern and reach levels of frightening. This bias could lead to wrongful arrests of people if police departments unwittingly use discriminatory facial recognition tools.
These AI bias examples only scratch the surface. Without the correct protocols in place, this flaw could significantly diminish the value of this game-changing tech.
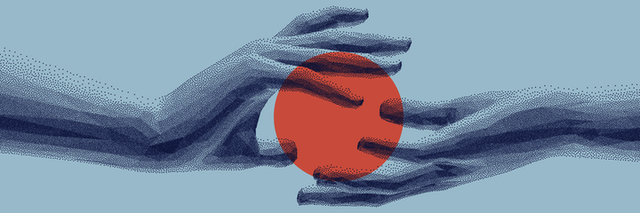
Reducing biases in AI
Organisations looking to offset AI biases can implement the following strategies:
- Data scientists must be taught about responsible AI and how unbiased organisational values should be ingrained into the model (or the model’s guardrails).
- Consumer transparency will help people grasp how AI algorithms make decisions and generate predictions. In other words, organisations utilising these tools must solve the “black box” problem impacting AI. This “black box” issue is best defined as customers seeing inputs and outputs but lacking awareness of how AI functions internally.
- A grievance process should be established by companies using AI. This way, individuals can engage in dialogue if they feel the technology has led to unjust treatment.
- Moreover, government regulation of AI can help better define and mitigate biases in AI. In doing so, regulatory bodies can clear up AI-related ambiguities and play a significant role in high-risk scenarios like credit, employment, surveillance, and education recommendations.
AI is here to stay–Pandora’s box is opened, and too much value is offered for people and businesses not to leverage this game-changing tech.
Conclusion: the jury is still out on unbiased AI
AI is here to stay–Pandora’s box is opened, and too much value is offered for people and businesses not to leverage this game-changing tech.
While AI will bring many positives, we can’t ignore its potential pitfalls, such as intrinsic biases within AI programming. Ultimately, people will always be the ones designing and implementing data into these systems–and humans can’t escape their biases.
Yes–steps can be taken to offset and reduce these biases.
Industry leaders can remain vigilant in detecting a lack of fairness and objectivity in the related tools. Still, full-on eradication seems like an unlikely feat. All we can do is take preventative steps and remain vigilant in ensuring AI treats everyone fairly and use it to make the world better.